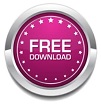

This may lead to indications that a model has not been trained sufficiently, has been subject to data poisoning (a form of back-door attack), or has learned to consider a spurious feature of the input that subject matter experts would not consider semantically related to the model’s output.īy observing saliency maps, we can characterize that model’s prediction behavior to understand how different conditions affect the model’s performance. While visual saliency maps do not provide a complete explanation of an AI model, they can provide insight into whether the model was considering something that semantically aligns, or does not align, with our human precepts for the task at hand.

Saliency maps are a form of visual explanation that indicate which input features were used by an AI model to generate its output decisions. Kitware Wins Honorable Mention Award for the xaitk-saliency package Pytorch’s Annual Hackathon 2021 Seeing is Believing: How Saliency Maps Work TPH-YOLOv5 (top) focuses on the head and feet of pedestrians, while CenterNet (bottom) focuses on the torso. (B) Despite having similar detections, the saliency maps corresponding to these detections reveal subtle differences in the input features used by the two models, e.g. (A) Both models produce similar high-confidence detections (shown with red bounding boxes). 2021b ) is shown in the top row and the CenterNet model ( Zhou et al. We computed object-specific saliency maps for two different models trained on the VisDrone aerial dataset ( Zhu et al. When augmented with a saliency algorithm, a visual explanation in the form of a saliency map is also produced, which can provide a user additional insight into how the algorithm generated its output.įigure 2 : Example saliency maps for comparing object detection models. Figure 1 shows an AI model pipeline, where xaitk-saliency algorithms can provide a form of XAI, and Figure 2 shows example saliency maps.įigure 1 : An AI model pipeline typically involves an algorithm that transforms input data into output predictions. Saliency maps are heat maps that highlight features in the input to an AI model that were significant in the AI model’s output predictions. This package provides a modular and extensible framework for invoking a class of XAI algorithms known as saliency maps. As part of this effort, we developed an open source python package within XAITK called xaitk-saliency. As a result, the XAITK has the potential to help human users better understand, appropriately trust, and effectively manage AI models. The XAITK contains a variety of tools and resources that help users, developers, and researchers understand complex AI models.
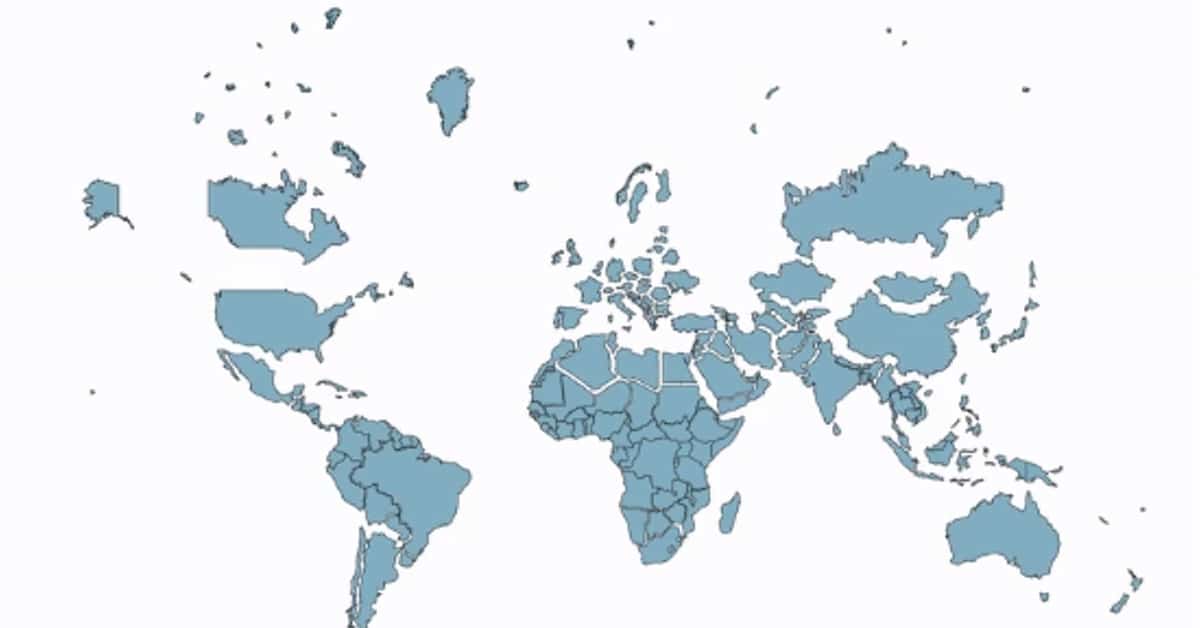
Kitware was thrilled to be involved in DARPA’s Explainable Artificial Intelligence (XAI) program and led the creation of the resulting Explainable AI Toolkit (XAITK). Despite the success of these AI models, their “black box” nature and lack of interpretability present a serious barrier to users, especially in domains with critical, high-stakes decisions such as healthcare, criminal justice, and autonomous driving. Research in artificial intelligence has seen significant progress over the past few years, spurring the increasing adoption of AI models in many real-world applications.
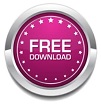